3D coke deformation recognition system by deep learning
Able to learn and estimate the coke strength after reaction (CSR) and deformation pattern
Overview
The gas permeability in the blast furnace deteriorates due to excessive coke
degradation during the low coke rate operation in order to reduce CO2 emission.
The coke strength after reaction (CSR) is a parameter to evaluate coke quality
and is used as an indicator of permeability in blast furnace operation.
However, CSR is an index that averages the high-temperature deformation
behavior of each cokes, and can’t estimate the complex deformation behavior of
individual coke particles. Existing method for flow phenomena visualization and
clogging prediction using kinetic model is computationally demanding and limited
in the ability to analyze stochastic deformation behavior in a scaling manner.
This invention applied deep learning using Deep Neural Network (DNN), which
is a typical AI method, to the coke 3D deformation process. Machine learning
was performed to estimate of the deformation process using DNN. As shown in
fig.1, the loss function (deviation from the “estimated value”) decreased with the
number of learning sessions, and the recognition accuracy shows over 97%. This
indicates that DNN can classify the deformation of every CSR accurately and
reduce the computational load for coke deformation prediction remarkably.
Therefore, machine learning can easily recognize the coke 3D shape
deformation which is difficult to recognize intuitively.
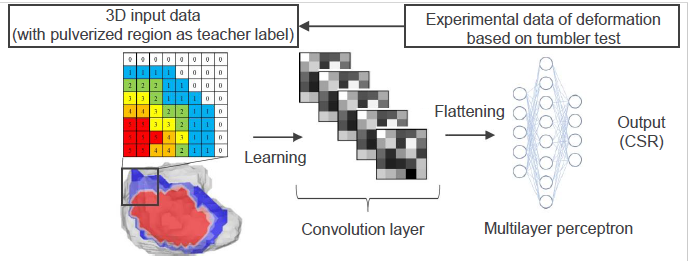
Over 97% CSR recognition accuracy by learning from experimental data
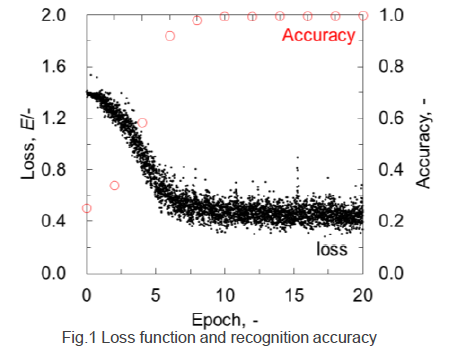
Product Application
・Periodical measurement of coke deformation pattern based on CSR value
in a production site
・A simple blockage estimation system of filling layer for coke mixing
process
・Creation of boundary condition data for kinetic model
Related Works
[1] Natsui, S., Hirai, A., Terui, K., Kashihara, Y., Murao, A., Miki, Y., & Nogami, H.
(2021). Method for Simulating Gas Permeability of a Coke Bed Including Fines Based
on 3D Imaging on the Coke Particle Morphology. ISIJ International, Vol. 61 (2021),
1814-1825
[2] Tammina, S. Transfer learning using VGG-16 with deep convolutional neural
network for classifying images; Int J. Sci. Res. Public., 9(10), (2019), 143-150.
IP Data
IP No. : JP2021-098241
Inventor : NATSUI Shungo, NOGAMI Hiroshi, HIRAI Azuma
keyword : Measurement, Machine, Control, Environment, Energy